Machine Learning and Deep Reinforcement Learning
I'm a fourth year student in Oxford with Shimon Whiteson at the Whirl-Group, mainly interested in deep reinforcement learning. Recently, I was lucky to have worked with Sam Devlin (at MSR Cambridge) and Nicolas Heess (at Deepmind) during two internships. Originally, my background is in Physics and Economics, both at the University of Munich (LMU), as well as Technology Management.
Google Scholar | Twitter | Linkedin | Github | Email
Research
My work focusses on improving transferability and generalization capabilities of reinforcement learning agents by leveraging ideas from hierarchical reinforcement learning, variational autoencoders and information theory. I'm most exited about deepening our understanding of RL and neural networks and developing new methods based on those insights.
Below is a list of selected papers. For a full list, please see my Google Scholar profile.
The Impact of Non-stationarity on Generalization in Deep Reinforcement Learning
M. Igl, G. Farquhar, J. Luketina, W. Boehmer, S. Whiteson | arXiv | code
Non-stationarity arises in Reinforcement Learning (RL) even in stationary environments, for example because we collect data using a constantly changing policy. In this work, we investigate how this affects generalization in RL and propose a new method, called Iterated Relearning (ITER), to improve generalization.
Generalization in Reinforcement Learning with Selective Noise Injection and Information Bottleneck
Igl M, Ciosek K, Li Y, Tschiatschek S, Zhang C, Devlin S, Hofmann K NeurIPS 2019 | arXiv | code
We explore the idea of using stochastic regularization, in particular the idea of an information bottleneck (as implemented by the DVIB) in the agent architecture to improve generalization to previously unseen levels in the Multiroom and Coinrun environments. To make it work well, we propose Selective Noise Injection to trade off regularization with training stability. The Gifs below show example rollouts of our agent (left) vs. previous state of the art (right) on unseen levels.
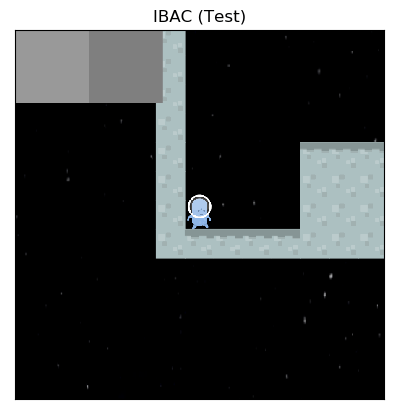
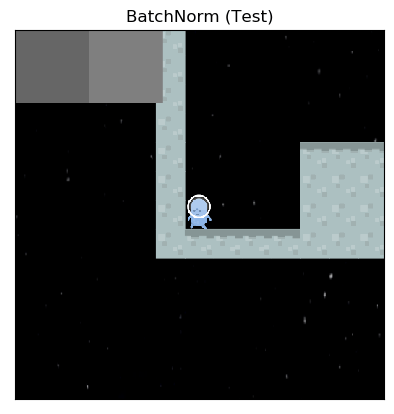
Multitask Soft Option Learning
Igl, M., Gambardella, A., He, J., Nardelli, N., Siddharth, N., Böhmer, W., & Whiteson, S. arXiv
We combine ideas from Planning as Inference and hierarchical latent variable models to learn temporally extended skills (called options). Given a set of different tasks, our approach allows to extract skills which are most useful across the range of tasks, i.e. most re-usable. Furthermore, we propose the idea of soft options, which allows to successfully apply previously learned skills, even in settings in which they are no longer optimal.
Deep Variational Reinforcement Learning (DVRL) for POMDPs
Igl, M., Zintgraf, L., Le, T. A., Wood, F., & Whiteson, S. ICML 2018 | arXiv | code
If the environment is only partially observable (which is typically the case in the real world), the agent has to reason about the possible underlying states of the world. To do so better, we propose Deep Variational Reinforcement Learning (DVRL) which learns a model of the world concurrently with the RL training and uses it to perform inference, i.e. to compute the agent's belief about its current situation.
Auto-Encoding Sequential Monte Carlo (AESMC)
Le, T. A., Igl, M., Rainforth, T., Jin, T., & Wood, F. ICLR 2018 | arXiv
We propose to combine Variational Autoencoders (VAEs) with Sequential Monte Carlo (SMC): When using VAEs for time-series data, using only one Monte Carlo sample can result in extremely high variance. Even using multiple samples with Importance Weighting might not be sufficient. Instead, we propose to use SMC, which uses clever resampling for variance reduction.